What this project is about and how to conquer it
Rui Qiu
Updated on 2021-09-16.
Early thoughts
People have always shown interests in sports, data, and those simulation games putting both together. Behind the intensity, the competitiveness, the joy of triumph, data play an essential role in shaping the modern boundary of sports. Just like in other industries, data is used as reflections and predictions at the same time. What fascinates people the most is that data provide a new perspective to appreciate games. For example, there might be some memorable moments when talking about a particular player's career, but collectively, is that overall impression about a player accurate? People might find ourselves in a position close to ignorance.
So in the portfolio, the topic selection is more like a loose collection of sports analytics applications. Because the data sources vary immensely, it is likely that no profound conclusions, like "how data would change the sports industry" or "what are the golden standards of being a basketball G.O.A.T. (the Greatest Of All Time)", will be drawn in the end. Instead, this is more or less like a showcase of what can be done when a fan stands at the crossroad of sports and data.
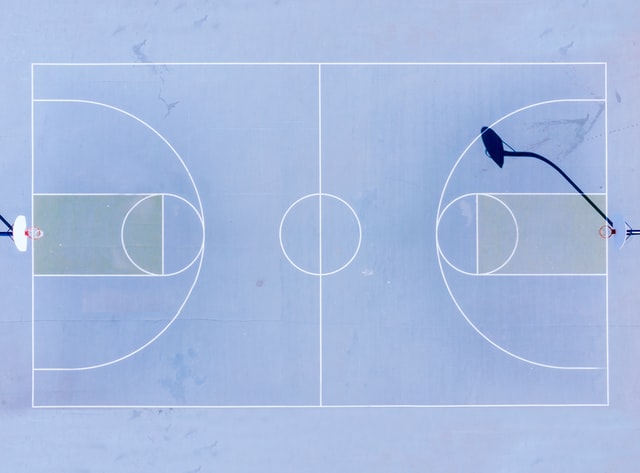
The sport of interest in this portfolio will be basketball because comparatively speaking, it has the most accessible open data.
An ever-updating roaster of questions
Ok, folks. Let's talk about the game plan.
The coverage of these questions ranges from tactics, performance to some off-court factors like social network interactions, etc.
Some rough plans about how to digest and answer these questions will be listed below as well.
The original twelve questions raised are:
-
Expected points: How to quantify the expected points from one shot? Inspired by the expected goal metric in soccer, is it possible to apply that in basketball and make it more sensible than the shot percentage?
-
Expected threats: Based on "expected points," is it possible to quantify a player's offensive contribution with a dynamic "expected threat" concept? Or how to quantify such metric when the player is not taking the shot, a.k.a. playing off-ball?
-
Clutch moment: When the clock is ticking, who is your most trustworthy ball handler, and who takes the last shot?
-
Player interactions: How do players interact with each other off-court? Do teammates/ex-teammates interact the most?
-
Social life vs. game plays: What's the relation (if any) between players' social media exposure and game performances? The more a player enjoys life, the worse he plays the next day or vice versa? This one is heavily influenced by an old Reddit post1 illustrating James Harden's game night performances vs. the overall strip club rating of the city he plays against that night.
-
Off-season topics: What are basketball fans' favorite topics in the off-season? Another social network-related question to raise.
-
Myths of power ranking: How to present teams' power ranking to a casual sports fan? ELO is an incredible invention, but is it good enough?
-
King of the off-season: Who's the king of the off-season? Another off-season secret. Some players choose to enjoy their lives, hanging out with friends, families; some go overseas for commercial reasons. But there can be only one king to rule it all.
-
Sixth man: Sixth man, or a hidden blade? The league always favors the non-starter with the most ppg in a Sixth Man voting. It sounds like a "Best Bench Offensive Player Award" instead.
-
Board man vs max contract: Board man gets paid? Is that true? It's a catchphrase from 2019 NBA champion Kawhi Leonard when he grabbed a rebound in a playoff game. But did all those rebounding leaders get paid in the reality?
-
Taco Tuesday: Does LeBron James revitalize the term Taco Tuesday? The 36-year-old Los Angeles Laker star has been famous for sharing his weekly taco dinners on social media since 2019. But does that matter? Does the former cause the latter?
-
Unlimited range: Who is the deadliest sniper? Back to a genuine basketball question. It is no secret that who the best 3-pt shooter in the league is The real question is, who is the most accurate mad man from downtown? Dame Dollar? Chef Curry? Imagine if NBA introduces the rule of a 4-point play. What's going to happen then?
However, due to the requirements of each sections of this portfolio, the actual ten questions that have been (partially) answered are:
-
How to visualize thousands of shot attempts effectively?
-
How to cluster NBA players by their overall statistics?
-
If new players are introduced to the game, can we put them in the correct cluster?
-
Is it possible to cluster news by categories so that any piece of future news can be categorized correctly?
-
Is is possible to expose some interesting rules in a player’s shot selection?
-
Can we actually predict a player’s shooting result based on some statistics from the offensive team with a tree-based model?
-
Can we predict the upvote status of an r/nba Reddit thread solely based on its title?
-
Can we predict a player’s shooting result based on more statistics including both offensive and defensive ends with another model?
-
Can we do the same to predict the popularity (other than upvote ratio) of a Reddit thread?
-
Can we visually compare the overall team shot attempt preferences?
Demo
Here is a demo of embedded Observable notebook.
Stack
Tech stack and resources used in this portfolio
Resources
This list of useful resources/references will be updated.
- carbon for code snippet screenshots.
- Poet for tweet screenshots.
- Feather icons.
- Unsplash for stock images.
- Design inspired by Noah Raskin and Tania Rascia.
- A Complete Guide to Flexbox
- Mining Twitter Data with Python Part 1: Collecting Data
- Tufte CSS
- JavaScript for R
- Prettier & Beautify
- Tweepy Documentation
- RedditExtractor
1. I analyzed James Harden's performance in every NBA city to see if there is a correlation between his box score and the city's average strip club rating. (reddit.com)↩